Comprehensive Guide to Descriptive Statistics
Descriptive statistics play a crucial role in the field of data analysis. They provide simple summaries about the sample and the measures, enabling us to understand and interpret data effectively. At Ivyleagueassignmenthelp, we delve into the various aspects of descriptive statistics, covering measures of central tendency, variability, data visualization techniques, and more.
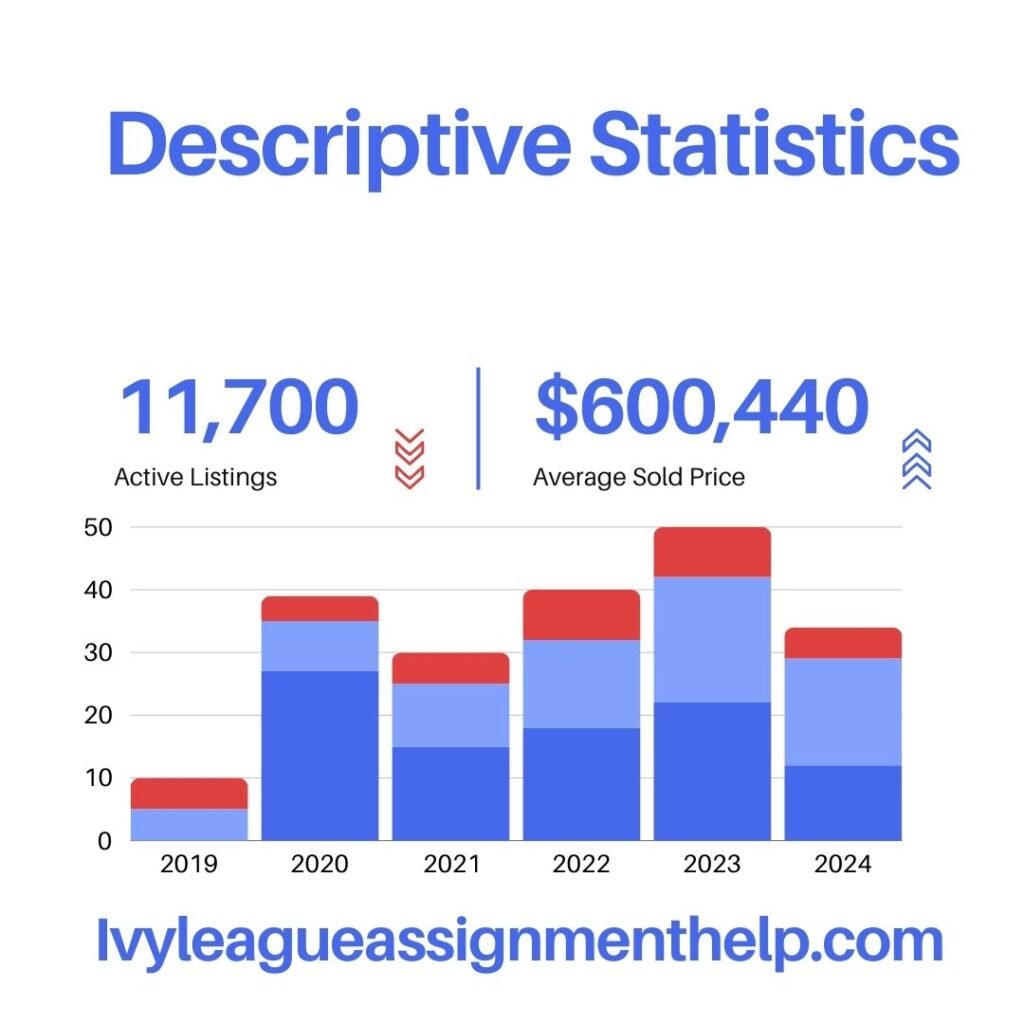
Understanding Descriptive Statistics
What are Descriptive Statistics?
Descriptive statistics are statistical methods that describe and summarize data. Unlike inferential statistics, which seek to make predictions or inferences about a population based on a sample, descriptive statistics aim to present the features of a dataset succinctly and meaningfully.
Importance of Descriptive Statistics
Descriptive statistics are fundamental because they provide a way to simplify large amounts of data in a sensible manner. They help organize data and identify patterns and trends, making the data more understandable.
Measures of Central Tendency
Mean
The mean, often referred to as the average, is calculated by adding all the data points together and then dividing by the number of data points. It provides a central value representing the data set’s overall distribution. The mean is sensitive to extreme values (outliers), which can skew the result.
Example:
Calculate the mean of the values below:
23,43,45,34,45,52,33,45, and 27
Mean (x) = [latex]\frac{{\displaystyle\sum_{}^{}}x}n[/latex]
x=[latex]\frac{23+43+45+34+45+52+33+45+27}9[/latex]
x = 38.56
Median
The median is the middle value when data points are ordered from least to greatest. If there is an even number of observations, the median is the average of the two middle numbers.
The mean, often referred to as the average, is calculated by adding all the data points together and then dividing by the number of data points. It provides a central value that represents the overall distribution of the data set. The mean is sensitive to extreme values (outliers), which can skew the result.
Example:
23,43,45,34,45,52,33,45, and 27
From the values, we can calculate the median.
23,27,33,34,43, 45,45,45, 52
From this, median = 43
Mode
The mode is the value that occurs most frequently in a data set. A data set may have one mode, more than one mode, or no mode at all if no number repeats. The mode is handy for categorical data where we wish to know the most common category.
23,43,45,34,45,52,33,45, and 27
From the figures, the number that appears repeatedly is 45.
Therefore, the mode = 45
Measures of Variability
Range
The range is the difference between the highest and lowest values in a dataset. It provides a measure of how spread out the values are.
Variance
Variance measures the average degree to which each point differs from the mean. It is calculated as the average of the squared differences from the mean.
Standard Deviation
Standard deviation is the square root of the variance and provides a measure of the average distance from the mean. It is a commonly used measure of variability.
Interquartile Range
The interquartile range (IQR) measures the range within which the central 50% of values fall, calculated as the difference between the first and third quartiles.
Data Distribution
Frequency Distribution
Frequency distribution shows how often each different value in a set of data occurs. It helps in understanding the shape and spread of the data.
Normal Distribution
Normal distribution, also known as the bell curve, is a probability distribution that is symmetrical around the mean, indicating that data near the mean are more frequent in occurrence than data far from the mean.
Skewness and Kurtosis
Skewness measures the asymmetry of the data distribution. Kurtosis measures the “tailedness” of the data distribution. Both are important in understanding the shape of the data distribution.
Data Visualization Techniques
Histograms
Histograms are graphical representations that organize a group of data points into user-specified ranges. They show the distribution of data over a continuous interval.
Box Plots
Box plots, or box-and-whisker plots, display the distribution of data based on a five-number summary: minimum, first quartile, median, third quartile, and maximum.
Bar Charts
Bar charts represent categorical data with rectangular bars. Each bar’s height is proportional to the value it represents.
Pie Charts
Pie charts are circular charts divided into sectors, each representing a proportion of the whole. They are useful for showing relative proportions of different categories.
Descriptive vs. Inferential Statistics
Key Differences
Descriptive statistics summarize and describe data, whereas inferential statistics use a sample of data to make inferences about the larger population.
When to Use Each
Descriptive statistics are used when the goal is to describe the data at hand. Inferential statistics are used when we want to draw conclusions that extend beyond the immediate data alone.
Feature/Aspect | Descriptive Statistics | Inferential Statistics |
---|---|---|
Definition | Summarizes and describes the features of a dataset. | Draws conclusions and makes predictions based on data. |
Purpose | Provides a summary of the data collected. | Makes inferences about the population from sample data. |
Examples | Mean, median, mode, range, variance, standard deviation. | Hypothesis testing, confidence intervals, regression analysis. |
Data Presentation | Tables, graphs, charts (e.g., bar charts, histograms). | Probability statements, statistical tests (e.g., t-tests). |
Scope | Limited to the data at hand. | Extends beyond the available data to make generalizations. |
Tools/Techniques | Measures of central tendency, measures of dispersion. | Sampling methods, probability theory, estimation techniques. |
Underlying Assumption | No assumptions about the data distribution. | Assumes the sample represents the population. |
Complexity | Generally simpler and more straightforward. | Often more complex and involves deeper statistical theory. |
Output | The initial stage of data analysis to understand the data. | Probabilities, p-values, confidence intervals, predictions. |
Usage | The later stage is to test hypotheses and make predictions. | The initial stage of data analysis is to understand the data. |
This comparison outlines the key differences between Descriptive and Inferential Statistics, highlighting their respective roles and techniques in data analysis.
Applications of Descriptive Statistics
In Business
Businesses use descriptive statistics to make informed decisions by summarizing sales data, customer feedback, and market trends.
In Education
In education, descriptive statistics summarize student performance, assess learning outcomes, and improve educational strategies.
In Healthcare
Healthcare professionals use descriptive statistics to understand patient data, evaluate treatment effectiveness, and improve patient care.
Common Misconceptions
Misunderstanding of Central Tendency
A common misconception is that the mean is always the best measure of central tendency. In skewed distributions, the median can be more informative.
Confusion with Inferential Statistics
Many confuse descriptive statistics with inferential statistics. Descriptive statistics describe data; inferential statistics use data to infer conclusions about a population.
Statistical Software for Descriptive Analysis
SPSS
SPSS (Statistical Package for the Social Sciences) is widely used for complex statistical data analysis. It offers robust tools for descriptive statistics.
R
R is a powerful open-source programming language and software environment for statistical computing and graphics, widely used among statisticians and data miners.
Python
Python, with libraries like Pandas and NumPy, provides extensive capabilities for performing descriptive statistical analysis and data manipulation.
Advanced Topics in Descriptive Statistics
Multivariate Descriptive Statistics
Multivariate descriptive statistics analyze more than two variables to understand relationships and patterns in complex data sets.
Descriptive Statistics for Categorical Data
Descriptive statistics can also summarize categorical data, using frequency counts and proportions to provide insights.
Descriptive vs. Predictive Analytics
Descriptive analytics focuses on summarizing historical data, while predictive analytics uses historical data to make predictions about future events.
Case Studies
Business Case Study
A retail company uses descriptive statistics to analyze customer purchasing patterns, leading to more targeted marketing strategies and increased sales.
Educational Research Case Study
An educational institution uses descriptive statistics to evaluate student performance data, identifying areas for curriculum improvement.
Healthcare Data Analysis Case Study
A hospital uses descriptive statistics to monitor patient recovery rates, helping to optimize treatment protocols and improve patient outcomes.
FAQs
What is the difference between mean and median?
The mean is the average of all data points, while the median is the middle value when the data points are arranged in order. The median is less affected by extreme values.
Why is standard deviation important?
Standard deviation measures the spread of data points around the mean. It helps in understanding how much variation exists from the average.
How do you interpret a box plot?
A box plot shows the distribution of data based on a five-number summary. The box represents the interquartile range, and the line inside the box is the median. The “whiskers” represent the range outside the interquartile range.
What is the role of skewness in data analysis?
Skewness indicates the asymmetry of the data distribution. Positive skewness means the data are skewed to the right, while negative skewness means the data are skewed to the left.
How can descriptive statistics be used in real life?
Descriptive statistics are used in various fields like business, education, and healthcare to summarize and make sense of large data sets, helping to inform decisions and strategies.
What software is best for descriptive statistics?
SPSS, R, and Python are all excellent choices for performing descriptive statistical analysis, each with its own strengths and capabilities.
Can you be more specific about the content of your article? After reading it, I still have some doubts. Hope you can help me.